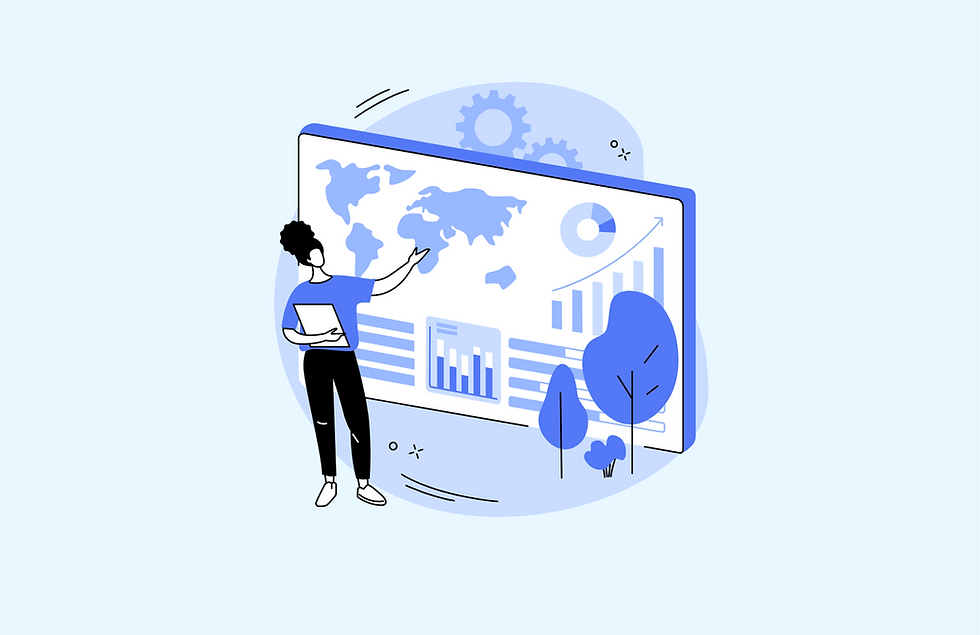
Navigating the complex world of data is like navigating uncharted territory. Picture standing at the crossroads of raw information, potential insights, and untapped opportunities. Each data point is hidden gem, poised to be discovered, polished, and transformed into a beacon of wisdom.
Welcome to the dynamic world of data observability. In this guide, we'll explore data observability, equipping you with strategies to illuminate the invisible, extract the meaningful and capitalize on powerful insights. So, thighten your boots and embark on a journey that will reshape how you perceive and harness the potential of your data.
What Is Data Observability?
At its core, data observability transcends mere data collection and storage. It’s about deeply understanding and deriving actionable insights from it.
Imagine your data landscape as a bustling city with countless streams of information flowing through intricate networks like busy streets. Data observability is the panoramic view that enables you to peer into every nook and cranny of this cityscape, spotting anomalies, patterns, and opportunities that might otherwise remain hidden. It’s your guiding compass in this data maze, illuminating the path to informed decisions, trend predictions, and the untold stories hidden within your data.
The Importance of Data Observability
Data observability sheds light on complicated data environments, empowering data management teams to craft robust and reliable data sets and pipelines. 90% of IT professionals believe observability is important and strategic to their business.
Unlike earlier data quality frameworks and tools that emerged alongside data warehousing, data observability extends beyond mere problem identification. Unlike the earlier data quality frameworks that emerged alongside data warehousing, data observability extends beyond mere problem identification. It lays the groundwork for reliability and resilience within the data environment.
This proactive approach, coupled with contextual insights, aids in the effective planning and prioritization of corrective actions. Ultimately, data observability minimizes data downtime by enabling real-time detection and evaluation of incidents, allowing immediate remedies. For instance, AI and machine learning-driven data observability platforms can promptly alert teams to anomalies and facilitate swift data restoration efforts.
Another important point of data observability is that it's a necessity for DataOps.
Data Observability and DataOps
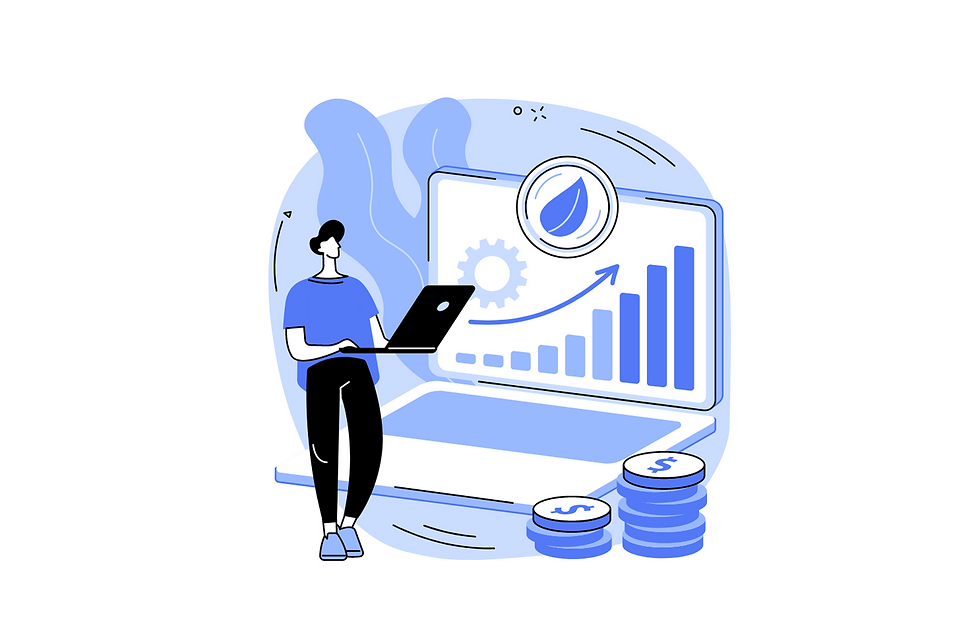
Data observability ensures data quality, detects issues, optimizes performance, and enables compliance. It provides real-time insights into data pipelines, facilitates issue resolution, and supports collaboration among teams, ultimately leading to efficient and reliable data operations.
Data observability offers a comprehensive range of capabilities to empower DataOps processes. It enables vigilant monitoring of data sets, whether they are stationary or in transit. Through this watchful lens, it ensures that data adheres to anticipated formats, types, and value ranges.
Additionally, data observability excels at anomaly detection, swiftly identifying schema modifications or abrupt shifts in data values that might signify underlying issues demanding attention. Furthermore, its prowess extends to the meticulous oversight and optimization of data pipelines, ensuring their optimal performance.
In essence, data observability becomes an indispensable asset in the DataOps arsenal, providing the tools to ensure data integrity, pinpoint anomalies, and enhance overall operational efficiency.
The Five Pillars of Data Observability
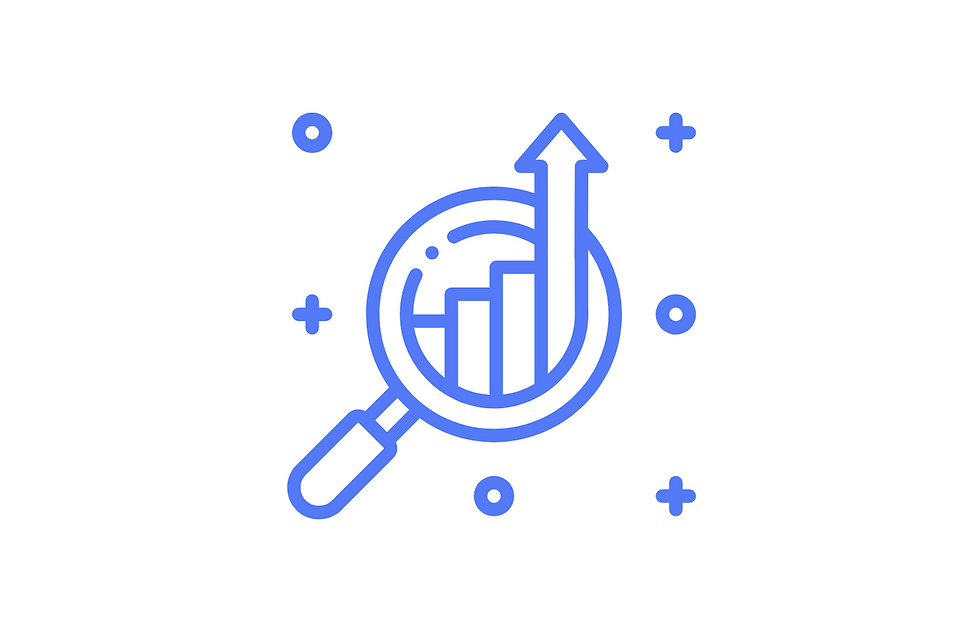
Here are the five pillars that form the foundation of data observability:
1- Freshness
Definition: This pillar focuses on the timeliness of data. It ensures that data is updated at expected intervals and is available for consumption without delays.
Importance: In today's fast-paced world, outdated data can lead to incorrect decisions. Monitoring data freshness ensures that stakeholders are working with the most recent and relevant data.
2- Volume
Definition: Volume observability pertains to monitoring the amount of data flowing through systems. It checks for unexpected spikes or drops in data volume.
Importance: Sudden changes in data volume can indicate issues like data loss, system outages, or unexpected surges in usage. Keeping an eye on volume helps in early detection of such anomalies.
3- Distribution
Definition: This pillar examines the distribution and variance of data. It ensures that data remains consistent and within expected ranges.
Importance: Anomalies in data distribution can hint at underlying issues like biased data sources or system errors. Monitoring distribution helps maintain data integrity.
4- Schema
Definition: It involves tracking changes in data structures or formats. It ensures that data adheres to a predefined schema and detects any deviations.
Importance: Unexpected schema changes can break downstream applications and analytics. By monitoring schema, organizations can ensure data compatibility and reduce integration issues.
5- Lineage
Definition: Data lineage provides a visual representation of data flow, showcasing its origin, transformations, and destinations.
Importance: Understanding data lineage helps in tracing errors back to their source, ensuring accountability, and providing clarity on how data is processed and consumed.
10 Data Observability Strategies for Powerful Insights
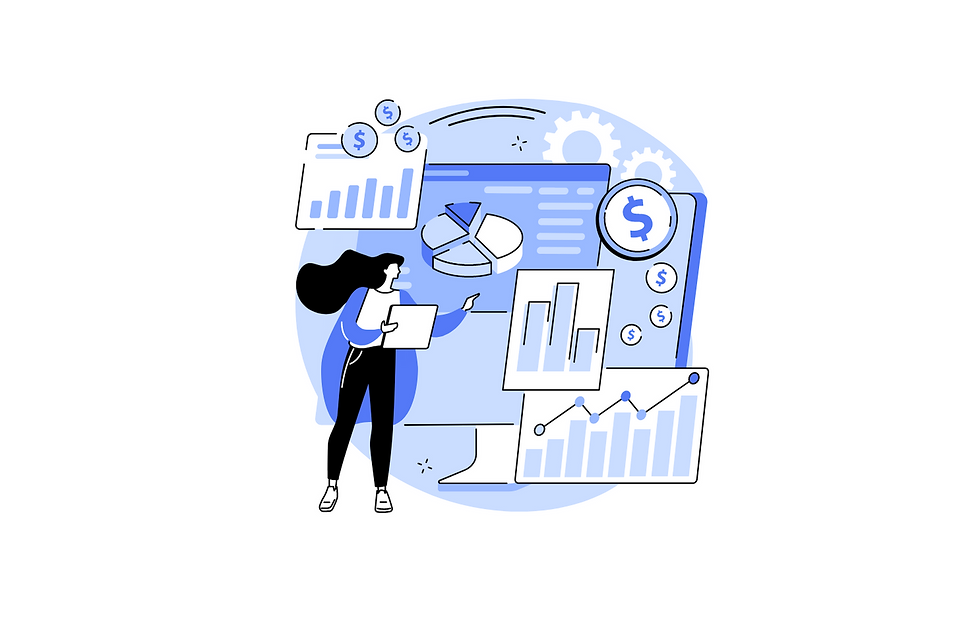
Just as in system observability, where we keep tabs on performance, errors, and logs, data observability focuses on metrics, logs, and traces of your data infrastructure. Adopting a robust data observability strategy empowers teams with insights to ensure data quality, reliability, and timeliness. Here are some strategies to help you achieve powerful insights through data observability:
1- Establish Clear Data Metrics
Lineage: Understand the journey of your data - where it originates, transformations it undergoes, and where it finally resides.
Freshness: Monitor how current or up-to-date your data is.
Volume: Track any unexpected spikes or drops in data volume.
Anomaly Detection: Use automated tools to detect anomalies in your datasets that might affect data accuracy.
2- Log Everything (But Be Smart About It)
Track data transformations, quality checks, failed jobs, and more.
Implement smart log rotation and retention policies to ensure older, less relevant logs don’t clutter the system.
3- Trace Data Through Its Lifecycle
Create a visual map or trace of data as it moves through different stages or systems. This helps in tracking down issues and understanding dependencies.
4- Implement Data Health Checks
Regularly check the quality of data at different stages. Use tools that offer automated quality checks and alert you in case of discrepancies.
5- Maintain Comprehensive Documentation
Document data schemas, transformations, data sources, and any known issues or quirks. Updated documentation helps teams swiftly address issues without starting from scratch.
6- Feedback Loops with Stakeholders
Regularly solicit feedback from data consumers, be it data analysts, business teams, or application developers. Their insights might alert you to observability gaps you hadn’t considered.
7- Invest in Specialized Tooling
There are many tools in the market that offer dedicated solutions for data observability. These tools can streamline the process, offering dashboards, alerts, and deep insights into your data landscape.
8- Use Metadata Effectively
Metadata, which is data about data, can be leveraged to understand aspects like data lineage, last updated timestamp, or source system. This enhances your data's observability by providing context.
9- Set Up Alerts for Critical Issues
Proactively monitor your data infrastructure. Set up automated alerts for issues like data pipeline failures, late data arrivals, or significant data quality issues.
10- Regularly Review and Refine
Data observability is not a one-time setup. Regularly review your metrics, logs, and traces to ensure they’re still relevant. As your system evolves, your observability strategies should adapt too.
By focusing on these strategies, organizations can ensure high quality and trustworthy data. As the adage goes, "You can't improve what you can't measure." With a robust data observability strategy, teams can effectively measure, monitor, and continuously refine their data infrastructure, leading to precise and timely insights.
Experience Advanced Data Observability With Datablast!
deeper insights and more precise data-driven decisions? Datablast is your go-to platform for comprehensive data observability. With us, you gain clarity on your data's health, reliability, and performance. It's not just about observing; it's about understanding and acting. Contact us today and redefine your data perspective!
Comments