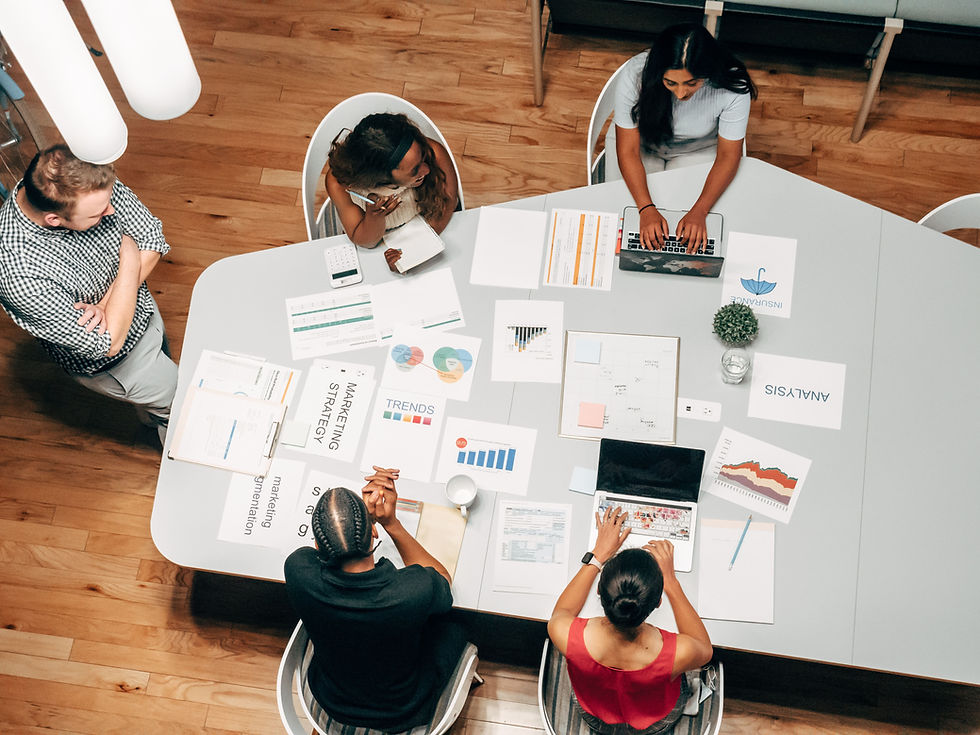
Today, retaining customers is more crucial than ever for sustained growth and success. Customer retention is the lifeblood of any business, as it directly influences revenue, profitability, and overall brand loyalty.
In this blog, we’ll explore how you can measure customer retention with cohort analysis, a data-driven technique that enables businesses to understand and measure customer retention effectively. Whether you're a seasoned business professional or new to the concept, this blog will provide you with valuable insights and practical steps to enhance your understanding.
Understanding Customer Retention
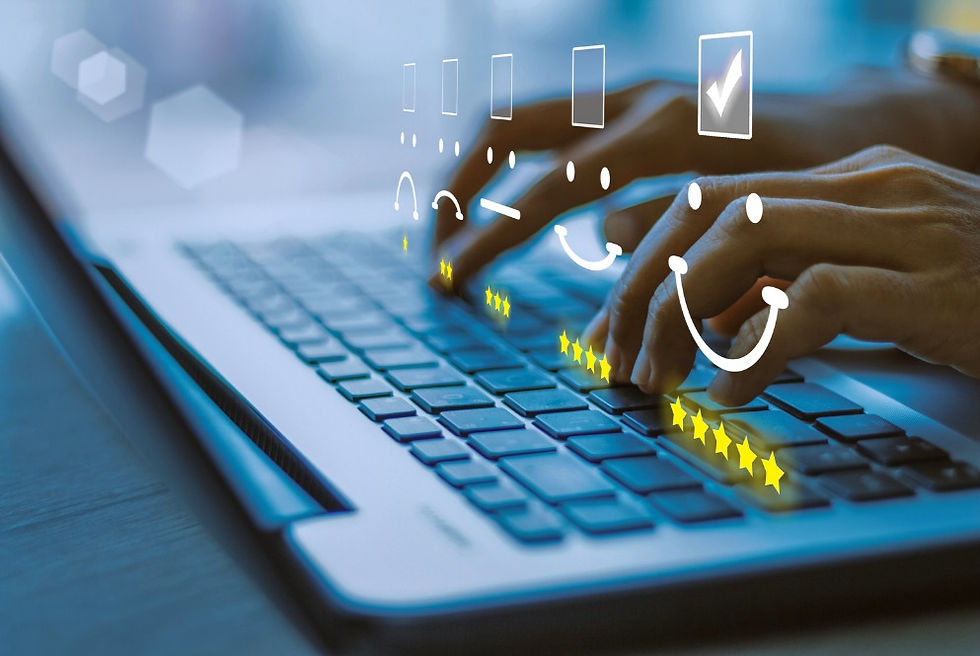
Customer retention is a critical aspect of any business's long-term success. It refers to the ability of a company to retain its existing customers over a specific period.
Instead of solely focusing on acquiring new customers, which can be more expensive and challenging, businesses must also prioritize retaining their current customer base. Customer retention plays a pivotal role in driving sustainable growth, increasing profitability, and fostering brand loyalty.
Understanding the key metrics and significance of customer retention is essential for businesses to develop effective strategies that nurture customer relationships and maximize customer lifetime value. These metrics include retention rate, churn rate, and repeat purchase rate.
Conducting Cohort Analysis for Customer Retention
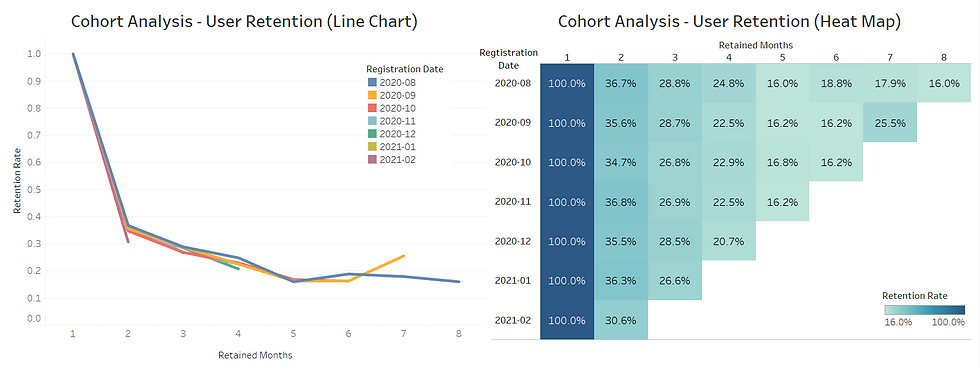
Conducting cohort analysis for customer retention is a vital practice that empowers businesses to gain valuable insights into customer behavior over time. By segmenting customers into cohorts based on common characteristics or acquisition periods, businesses can identify patterns in customer retention rates and make informed decisions to enhance customer loyalty.
The process involves organizing and analyzing data to calculate retention rates for each cohort, providing a clear understanding of how customer retention evolves within specific groups.
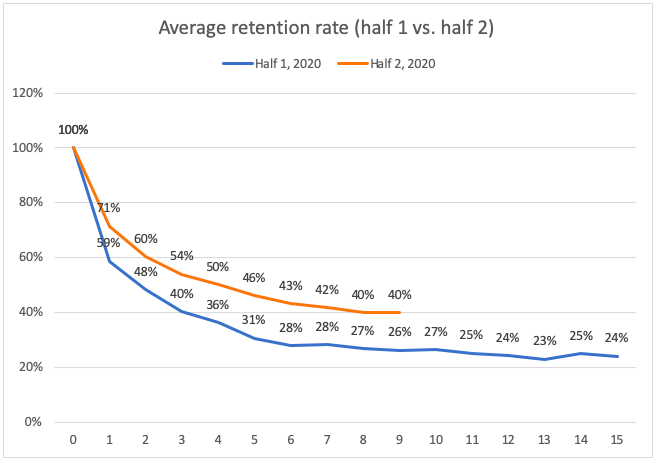
To have a clear understanding of what a cohort looks like, a combination of these three points can reveal a cohort:
Users with common but specific traits (e.g. premium users)
Users who act similarly (e.g. downgrade to a standard plan)
A defined period (e.g. in July)
Cohort Metrics
Cohort metrics are a powerful tool that enables businesses to track and analyze customer behavior over time. These metrics typically focus on key performance indicators such as user retention, engagement, and revenue generation.
Instead of settling for a simple metric like "users installed app in January," cohort metrics allow us to dig deeper, such as "IOS users who installed it in the US on June 17th," and compare this metric with similar data for April 15 for more comprehensive and meaningful analysis.
What can be the cohort metrics?
Share of users still active 3 months after their first activity
Share of users still subscribed 6 months after making their first purchase
Share of users who sign up for a free trial and convert into paying customers within 14 days after starting the trial
Utilizing these metrics allows us to gain deeper insights into customer interactions, make data-driven decisions, and optimize the customer experience. If we generalize about metrics, the denominator will usually be the size of the cohort. The denominator is the number of customers retained or some other metric such as total money spent.
Cohort Definition
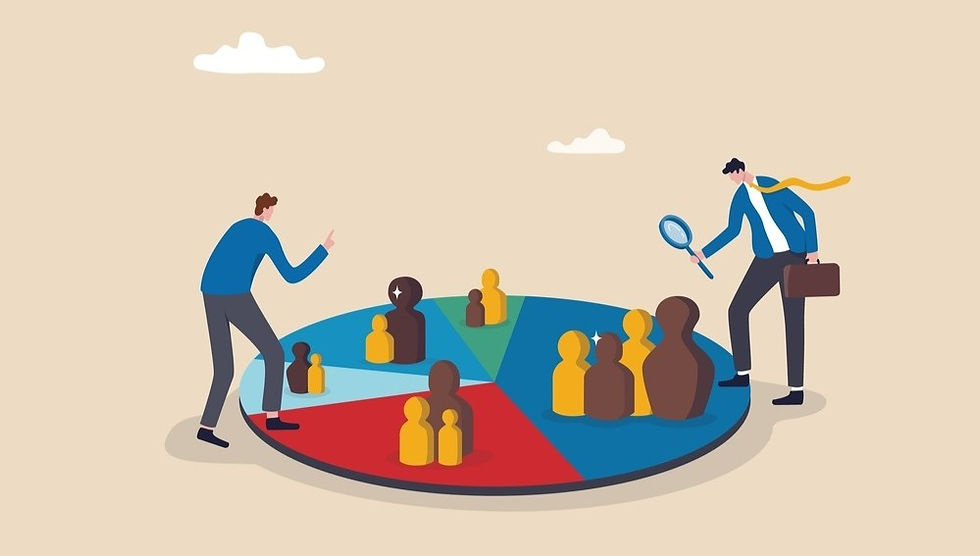
Defining cohorts is a fundamental step in cohort analysis, where groups of users sharing common characteristics or experiencing similar events are organized for in-depth analysis. Cohorts can be formed based on various attributes, such as the time of user acquisition, geographical location, or user behavior.
Defining cohorts allows companies to identify trends, track changes in user engagement, and measure the success of specific initiatives, ultimately aiding in strategic decision-making and optimizing customer retention efforts.
To make it a little more concrete:
Users who entered the website from Turkey via the web on July 25th
Users who entered the website from Turkey via phone on July 25th.
In this example, the cohort definition is based on user behavior and source on July 25th. The cohort consists of two groups: users who accessed the website from Turkey via the web and users who visited from Turkey via phone on that specific date.
For the web users, businesses can track metrics such as the average time spent on the site, the most visited pages, and the percentage of users who filled out forms and converted into customers. This data can reveal which pages or content are more engaging and effective in driving conversions.
Similarly, for phone users, the same metrics can be monitored to understand their browsing and conversion patterns. Additionally, businesses can compare the performance of web users with phone users to identify any differences in behavior and tailor their strategies accordingly. For example, if those coming from the web spend more time, we can make an inference that when entered from the phone, our mobile version might have a problem such as insufficient UI.
Additionally, you can address:
Return on Investment (ROI)
Customer Lifetime Value (LTV)
Customer Acquisition Cost (CAC)
Use Case: Examine how the launch of a new feature affects retention rates
Releasing a new version or introducing a new feature can significantly impact a business, setting new expectations and goals. Increased engagement is often a primary objective in such cases. Cohort analysis emerges as a tool to gain a clear understanding of user behavior and performance under these circumstances.
When conducting cohort analysis for this purpose, it's beneficial to include the install version as part of the cohort details. This addition allows you to discern whether the new version indeed delivered the expected results. Moreover, to assess the impact of the feature without a full-scale rollout, A/B testing can be employed. Running an A/B test involves measuring key metrics while defining the AB group as the main part of the cohort. This approach helps you evaluate the effectiveness of the feature in a controlled setting, offering valuable insights for refining strategies and achieving optimal results.
By leveraging cohort analysis and incorporating A/B testing, businesses can precisely evaluate the impact of new versions or features, and tailor their approach to enhance user engagement and overall success.
Gross & Net Retention
Gross retention and net retention are two different methods of measuring customer retention in a business.
Gross Retention
Gross retention, also known as gross churn, measures the percentage of existing customers who continue to remain customers within a specific time period, without taking into account any additional revenue generated from upsells, cross-sells, or expansion from existing customers. Additionally, a customer that churns and comes back later on another period won’t be counted as a retained customer. Instead, they can be included in a new cohort, in which month they come back.
In other words, it only considers customer retention without factoring in any revenue growth from the existing customer base. Gross retention provides a straightforward view of customer loyalty and how well a company is retaining its current customers.
Net Retention
Net retention, also known as net churn or net revenue retention, takes into account both customer retention and additional revenue generated from existing customers during the same time period.
It considers revenue lost due to churn (canceled subscriptions) as well as revenue gained from expansion (upselling, cross-selling, and customer upgrades) within the existing customer base. Unlike gross retention, here a churning customer will be considered retained when they return. Since Customer Acquisition Cost (CAC) considers only the first time when you acquire a customer, net retention values will be suitable to calculate your customer acquisition cost.
However, this method may not always be suitable for having insights about the returning customer, for example, if a churned customer comes back years later, you may not get a clear insight. In such cases, it would be more appropriate to use the gross retention method.
By comparing gross retention and net retention rates, businesses can gain insights into how effectively they are retaining customers and growing revenue from the existing customer base.
Interpreting Cohort Analysis Results
Once you have conducted the cohort analysis for customer retention, it's essential to interpret the results to derive meaningful insights and actionable conclusions.
Armed with the knowledge you gained in the previous steps, you can implement targeted strategies, improve customer engagement, and ultimately foster long-term relationships with your customer base.
Analyzing Retention Curves
The first step in interpreting cohort analysis results is to analyze the retention curves for each cohort. Retention curves visually represent the percentage of customers retained over time within a specific cohort. These curves provide valuable information about customer behavior, such as whether retention rates increase or decrease over time, and how different cohorts compare to one another.
Identifying Trends and Patterns
During the analysis, it's essential to identify any trends or patterns that emerge from the cohort data. Look for cohorts with high retention rates, which indicate successful customer retention strategies, and investigate what sets them apart. Conversely, pay attention to cohorts with declining retention rates, as they may signal potential issues or areas for improvement in your customer experience or engagement efforts.
Drawing Insights for Optimization
The primary goal of cohort analysis is to draw actionable insights to optimize customer retention. Based on the patterns and trends identified, you can develop strategies tailored to specific cohorts. For example, if a certain cohort exhibits excellent retention, consider replicating the factors that contribute to their loyalty across other cohorts. On the other hand, for underperforming cohorts, you can implement targeted interventions, such as personalized communication, loyalty rewards, or product enhancements, to boost retention rates.
However, cohort analysis is not a one-time exercise. Regularly updating and reanalyzing your cohort data will allow you to assess the effectiveness of your retention strategies and adapt them as needed. Moreover, tracking cohort behavior over time can help you spot long-term trends and understand the impact of changes in your business or marketing strategies on customer retention.
Want to Implement Cohort Analysis for Measuring Customer Retention? Meet Datablast
Datablast's expert data services and the powerful platform are here to help. Our comprehensive solutions, tailored to your unique needs, will transform your data into actionable insights that drive growth and success. Visit our website to contact us and start your journey towards data-driven excellence!
コメント