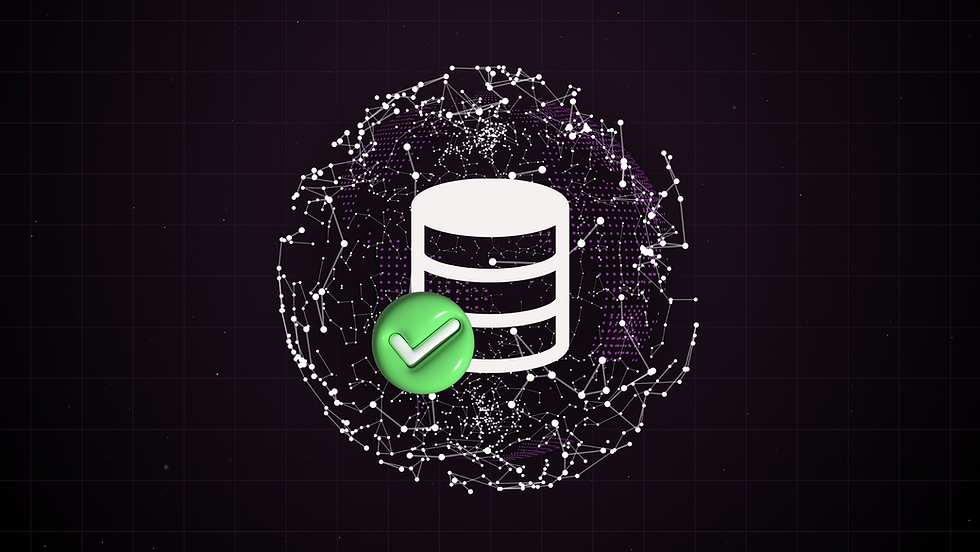
In 2024, the cornerstone of organizational success hinges on the integrity and reliability of data. As businesses increasingly rely on sophisticated algorithms and data, the margin for error narrows, making the pursuit of impeccable data quality a necessity. This blog post will take you on a comprehensive journey through data quality improvement, offering insights into proactive measures, advanced technologies, and best practices that ensure your data assets aren’t only accurate and consistent but also aligned with the dynamic demands of the future. Take this as a roadmap to transforming data quality from a potential vulnerability into a formidable competitive advantage, ensuring that your organization thrives in the data-centric era of 2024.
What Is Data Quality & Why Is It Crucial?
Data quality refers to the accuracy, completeness, reliability, and relevance of data within the context of its intended use. It’s a critical aspect of data management that ensures information is fit for making informed decisions, driving business strategies, and supporting operational processes. High-quality data is crucial because it directly impacts the effectiveness of analytics, the efficiency of business operations, and the integrity of insights derived from data. Poor data quality, on the other hand, can lead to misguided decisions, operational inefficiencies, and a significant loss of revenue. For instance, due to data migration and system issues, many health workers were overpaid, underpaid, or not paid at all by the Queensland Government. The debacle cost taxpayers an estimated AUD 1.2 billion. Therefore, maintaining high data quality is essential for organizations to remain competitive, compliant, and capable of delivering value.
7 Key Dimensions of Data Quality
The fundamentals of data quality revolve around several key dimensions that ensure the data is accurate, reliable, and useful. These dimensions include:
1. Accuracy: The data needs to be accurate, reflecting reality or coming from a reliable source. It should be error-free and convey the intended meaning clearly.
2. Completeness: All necessary data should be available and not missing any critical component. It should cover the required scope for its intended use.
3. Consistency: Maintain consistency throughout diverse datasets or databases, eliminating any contradictions or discrepancies. The data should seamlessly integrate with other relevant information.
4. Timeliness: Data needs to be up-to-date and available when needed. It should reflect the most current situation or information.
5. Reliability: Data should be collected and maintained in a manner that ensures its trustworthiness over time. It should be sourced from credible sources and maintained with integrity.
6. Relevance: Ensure that the data is applicable and useful for the context in which it’s used. It should meet the needs of its intended users or applications.
7. Accessibility: Data should be easily retrievable and usable by authorized users. It should be stored in a manner that facilitates easy access and analysis.
8. Uniqueness: Make sure that each data element is unique and not duplicated. This ensures clarity and efficiency in data management.
These dimensions collectively ensure that data is of high quality and can be effectively used for analytics, decision-making, and operational processes.
What Are the Best Practices for Data Quality Enhancement?
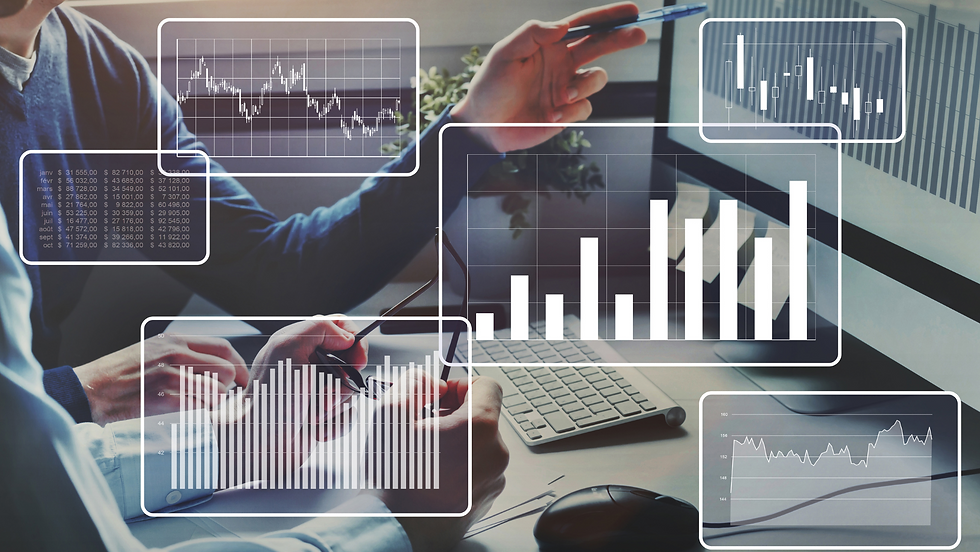
As the volume, variety, and velocity of data continue to expand, traditional methods of maintaining data quality are being supplemented and even replaced by more advanced techniques and technologies. Best practices for data quality enhancement encompass a range of strategies, from the initial data collection phase through to the ongoing management of data. Here, we explore the cutting-edge approaches and technologies that are setting new standards in the pursuit of pristine data quality.
Proactive Data Quality Measures
In 2024, it’s critical to be proactive about data quality. Why? Because so will your smart competitors. You might be thinking “What does being proactive ensure?” The answer is quite simple: It enables you to anticipate and prevent data quality issues for your business before it's too late. Emphasizing proactivity leads to a number of important outcomes, such as reducing disruptions in operations and ensuring data accuracy and consistency. For this reason, it shouldn’t be underestimated.
Automated Data Quality Management Systems
Automation stands at the forefront of modern data quality improvement strategies. Automated data quality management systems leverage artificial intelligence (AI) and machine learning (ML) algorithms to continuously monitor, clean, and enrich data without significant human intervention. These systems can identify and rectify errors, inconsistencies, and duplications in real-time, significantly reducing the time and resources traditionally required for data cleansing.
Predictive Data Quality
Predictive data quality utilizes machine learning models to anticipate potential data quality issues before they occur. By analyzing patterns and trends in the data, these models can predict anomalies, outliers, and errors, allowing organizations to proactively address these issues. This forward-looking approach helps in maintaining the integrity and reliability of data over time.
Proliferating Data Governance
Establishing comprehensive policies, procedures, and standards to manage and ensure the accuracy, completeness, consistency, and reliability of data across an organization is crucial. This approach emphasizes the importance of data as a strategic asset, requiring stewardship at every level of the organization to maintain its integrity. By implementing a robust data governance framework, you can enhance decision-making, comply with regulatory requirements, and improve operational efficiencies. This framework typically includes roles and responsibilities for data management, clear data quality metrics, and processes for data collection, storage, and access, thereby fostering a culture of accountability and continuous improvement in data quality.
Data Quality as a Service (DQaaS)
The rise of cloud computing has led to the emergence of Data Quality as a Service (DQaaS), offering data quality tools and services on a subscription basis. DQaaS provides businesses with access to sophisticated data quality solutions without the need for substantial upfront investment in infrastructure or software. This model supports scalability, flexibility, and access to the latest advancements in data quality technologies.
Following Data Quality Metrics and Dashboarding
Advanced data quality solutions now incorporate comprehensive metrics and dashboarding capabilities, providing organizations with real-time insights into the state of their data quality. These tools enable data managers to track key performance indicators (KPIs), identify trends in data quality issues, and measure the impact of data quality initiatives over time.
The advancement in techniques and technologies for data quality is transforming how organizations approach data management. If you adopt these innovative solutions as a business, you can ensure the reliability, accuracy, and integrity of your data, thereby enhancing your analytical capabilities and competitive edge.
What to Expect for Future Trends in Data Quality Management?
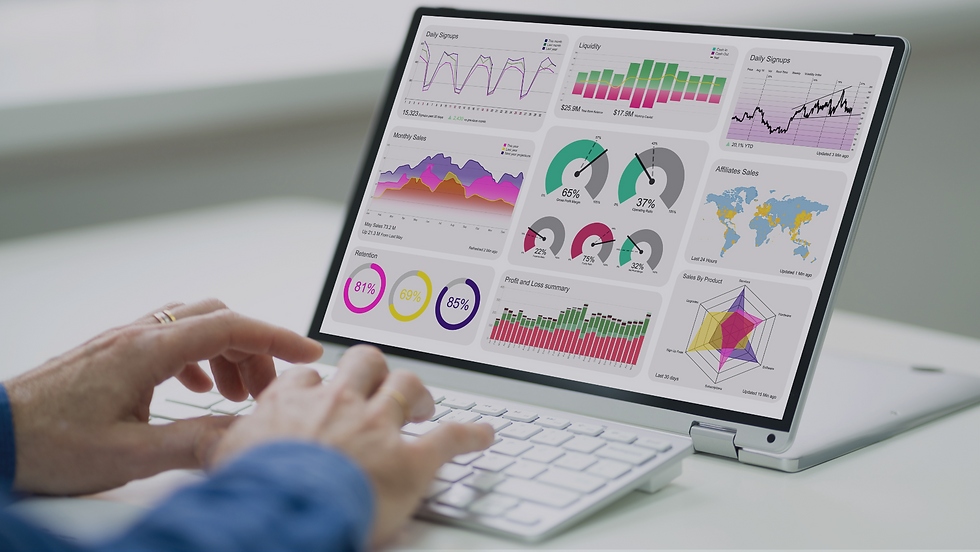
Future trends in data quality management (DQM) are poised to address the increasing complexity and volume of data. As organizations continue to recognize the critical role of high-quality data in predictive analytics and operational efficiency, there’s a clear shift towards more automated, intelligent, and integrated DQM solutions. Advanced technologies such as artificial intelligence (AI) and machine learning (ML) are at the forefront of this transformation, offering the potential to significantly enhance the accuracy, consistency, and speed of data quality processes. These technologies enable real-time data monitoring and validation, predictive data quality assessments, and automated correction of data anomalies, thereby reducing human error and improving overall data integrity.
Moreover, the integration of DQM practices into the broader data governance and management strategies is becoming increasingly important. Organizations are expected to adopt a more holistic approach to data quality, emphasizing the alignment of data quality initiatives with business objectives and regulatory requirements. This includes the development of comprehensive data quality frameworks that encompass data privacy, security, and compliance considerations. As data ecosystems become more complex and interconnected, the emphasis on collaborative, cross-functional teams equipped with sophisticated DQM tools and platforms will be critical in ensuring that data remains a reliable, strategic asset in the digital age.
Don't Leave Data Quality to Chance: Meet Datablast!
Leaving data quality to chance in 2024 is not enough to take your business to the next level. As Datablast, we transform complex data into actionable insights that fuel your business growth. Our comprehensive data pipeline system ensures that as your business scales, your data's complexity and volume become assets, not obstacles. Our dynamic quality checks prepare your organization for future challenges.
Datablast simplifies data management, offering scalable metrics architecture, a single source of truth (SSOT) for multiple insights and automated data pipelines for optimal efficiency. Contact us today to unlock your data's potential and ensure your data quality is never left to chance.
Comments